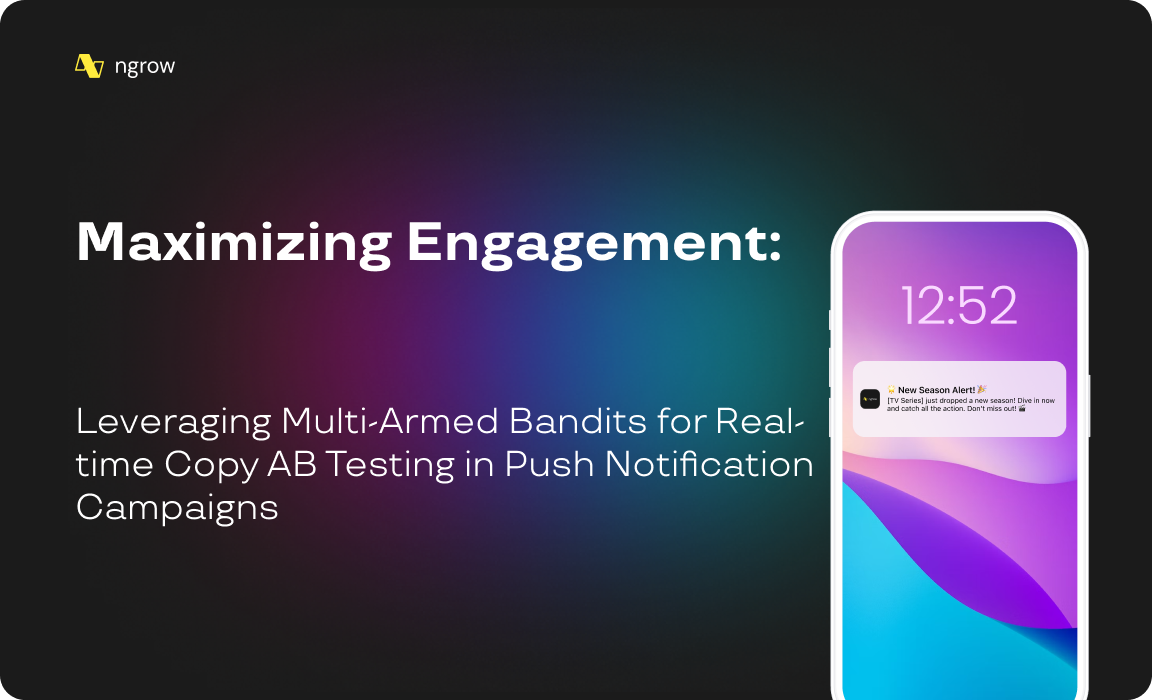
The world has moved from manual testing to automation. But even when we talk about automation, there are a few things that stick out - How much information is too much information? How do we get the ability to optimize campaign creatives for maximum engagement?
One innovative approach that has gained traction in recent years is the use of Multi-Armed Bandits for real-time copy AB testing in push notification campaigns. This technology offers a dynamic and efficient way to test and validate hundreds of creatives simultaneously, providing marketers with valuable insights to enhance their campaigns. Let's delve into the intricacies of this approach and explore its benefits for optimizing push notification campaigns.
Understanding Multi-Armed Bandits
First things first, what are multi-armed bandits?Multi-Armed Bandits is a machine learning algorithm that falls under the umbrella of reinforcement learning. The concept is derived from the analogy of a gambler facing multiple slot machines (bandits) with different payout probabilities. In the context of marketing, each "arm" represents a creative variant, and the algorithm dynamically allocates traffic to these variants based on their performance.
So to simplify this, it is a reinforcement learning technique used to balance exploration (trying new options) and exploitation (using the current best option) in decision-making processes. There are various MAB algorithms, such as ε-greedy, Upper Confidence Bound (UCB), and Thompson Sampling, which are used to optimize decisions in uncertain environments.MABs have wide-ranging applications in various fields, including advertising, healthcare, web optimization, dynamic pricing, network routing, and machine learning, due to their ability to balance exploration and exploitation effectively.
The Multi-Armed Bandit (MAB) problem is a classic reinforcement learning example where the objective is to pull the arms of a slot machine in sequence to maximize the total reward collected in the long run.
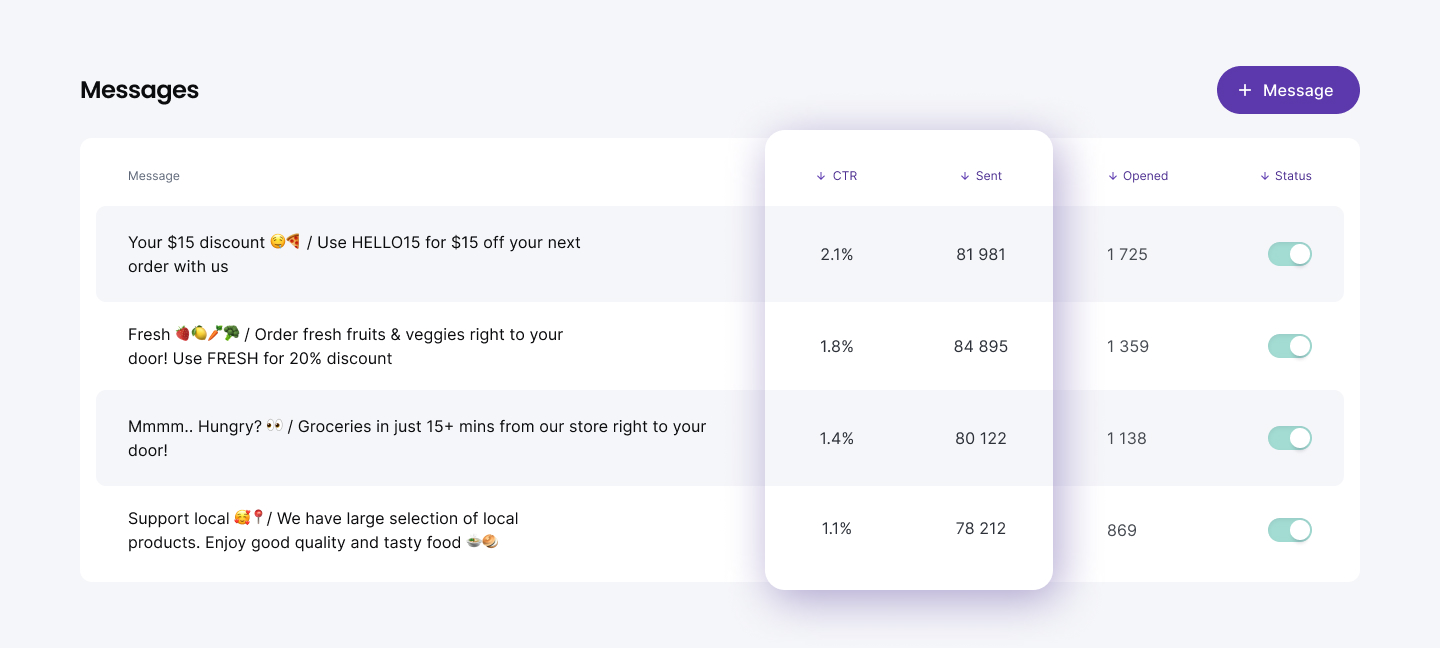
The Utility of Multi-Armed Bandits in Push Notification Campaigns
When dealing with a large volume of inputs such as push notifications, traditional A/B testing methods can be time-consuming and inefficient. Multi-Armed Bandits offer a more agile solution by continuously exploring new variants while exploiting the best-performing ones. This adaptive approach allows marketers to quickly identify high-performing creatives and allocate more traffic to them, leading to improved engagement rates and better conversions.
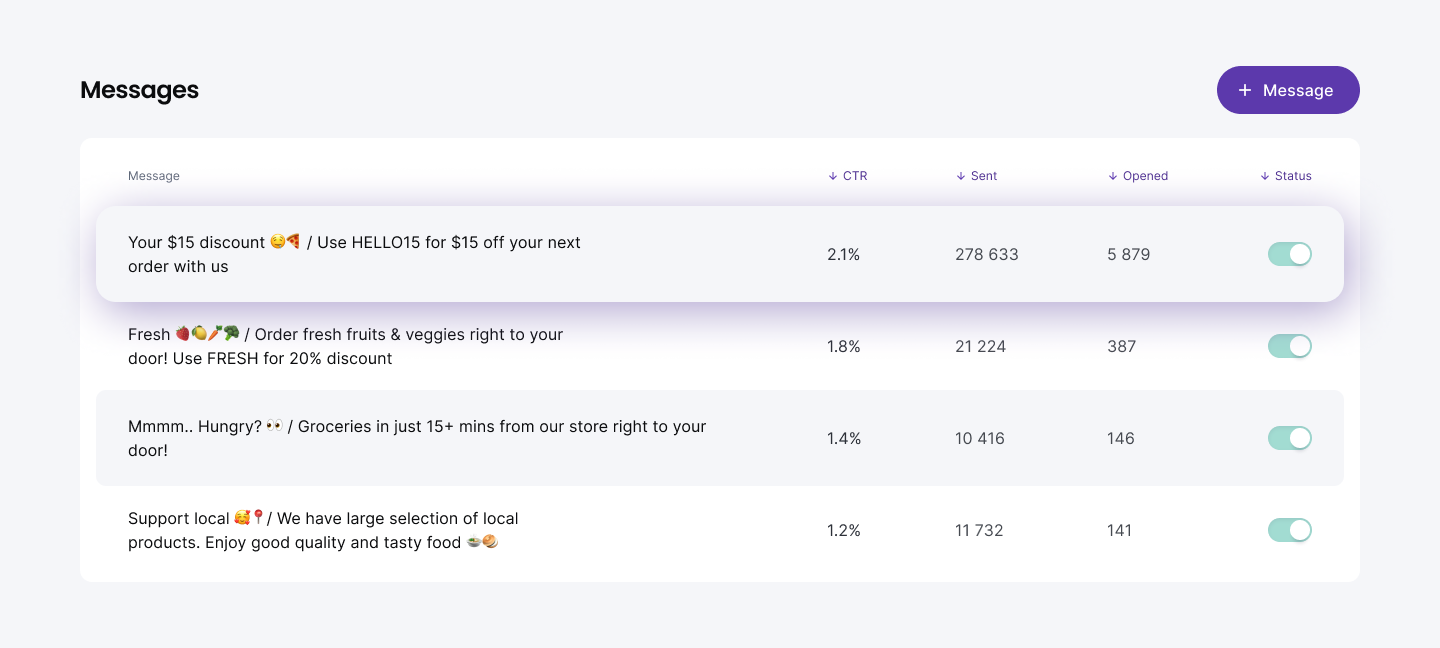
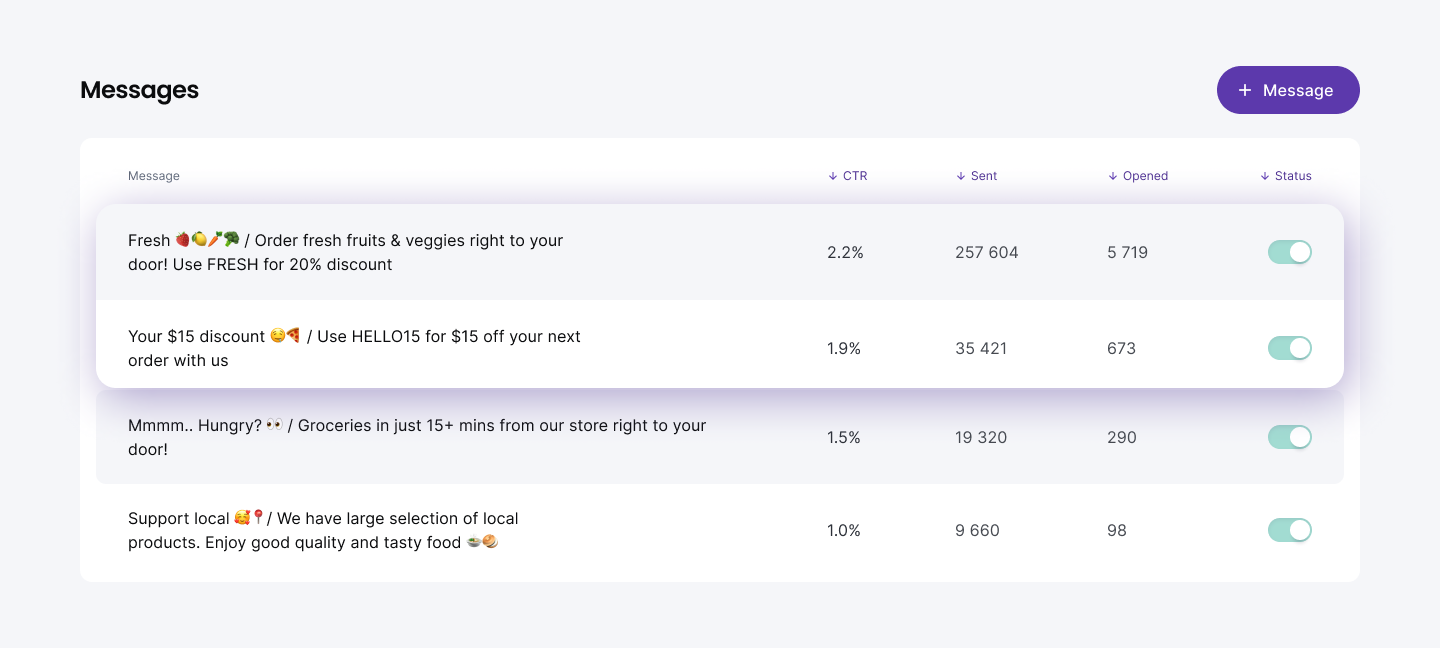
Facilitating Cohort-Based Decision Making
One of the key advantages of using Multi-Armed Bandits for push notification campaigns is its ability to facilitate cohort-based decision making.
By segmenting users into cohorts based on various attributes such as demographics, behavior, or preferences, marketers can tailor their messaging to specific audience segments.
The algorithm dynamically adjusts traffic allocation within each cohort, ensuring that the most relevant creatives are delivered to the right audience at the right time.
A case study to understand the use-case better is the Amma Case Study where ML engine uses a multi-armed bandits algorithm, which allows simultaneous testing of all push text variations and sorting them according to their efficiency.
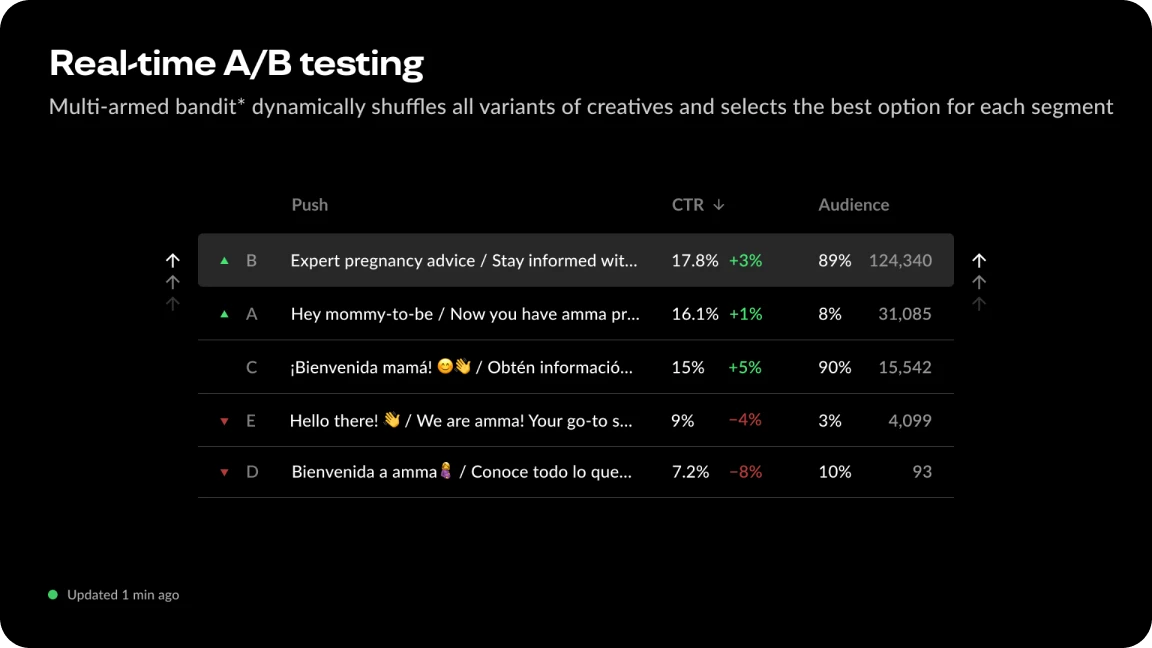
In conclusion, leveraging Multi-Armed Bandits for real-time copy AB testing in push notification campaigns offers marketers a powerful tool to optimize engagement and drive conversions. By harnessing the adaptive nature of this algorithm, marketers can efficiently test and validate a large number of creatives simultaneously, leading to data-driven insights that enhance campaign performance.
Embracing this technology enables marketers to stay agile in an ever-evolving digital landscape and deliver personalized experiences that resonate with their target audience.